PhD Supervisor
Professor
Department of Electronics and Electrical Engineering
IIT Guwahati - India
Phone: +91-361-2582507
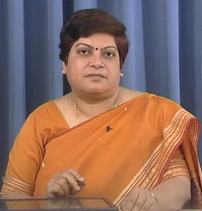
Courses taken during PhD
PhD date of joining - Sep 2020
Courses done (Sep 2020 - May 2021)
​
Cumulative Performance Index (CPI) - 9.80/10
​
-
Robust Control - Grade AA (6 credits)
-
Linear Systems Theory - Grade AA (6 credits)
-
Linear Algebra and Optimization- Grade AB (6 credits)
-
Modeling and Simulation of Dynamic Systems - Grade AA (6 credits)
-
Optimal Control - Grade AA (6 credits)
​
June 2021 - Comprehensive Viva completed.
December 2021 - State of the Art Seminar completed.
December 2022 - 1st Annual Progress Review Seminar completed.
December 2023 - 2nd Annual Progress Review Seminar scheduled.
Development of a Realtime Energy Management System for Electric Vehicles
As the population of India is increasing, the demand for adequate transportation facilities has also increased exponentially. To cater to such a huge market, Internal Combustion Engine (ICE) vehicles are predominantly produced and being purchased by people. ICE vehicles are creating pollution which is directly or indirectly affecting the environment and health of all living beings on earth. ICE vehicles emit pollutants which are not only causing diseases but also reducing the life expectancy of humans. The phenomenon of climate change is impacting the world and according to UN climate change report India will experience extreme weather events in the 21st century.
Electric Vehicles (EVs) are coming out as a suitable substitute to ICE vehicles to protect environment and human race. But the development of commercial EV has not been accelerated enough. There may be many reasons for it such as lack of infrastructure i.e. charging points in India, reluctance of Indian population towards a new technology, improper roads conditions, economic aspect and mindset of people etc.
There is a lot of scope of research in working and economics of EV in terms of populated countries like India or other Asian countries. The previous studies investigated different aspects of EV such as economic aspect, optimisation of EV size, Energy Management System (EMS) etc. However, these researches were conducted on typical drive cycles without taking the practical conditions prevailing in these countries into consideration. In order to minimise the impact on environment, the most populated countries should be the target and work should focus on shifting the population of these countries towards healthy choice for future.
​
In India, the road condition such as slope is varying after every 10m. For efficient working of vehicle these conditions play an important role. The previous studies focussed on the energy management system of EV for enhancing the life cycle of batteries considering the factors such as State of Charge (SOC) of Supercapacitor (SC), SOC of battery, power consumed by sources etc but neglected the conditions of roads.
These factors motivated me to design a controller which can efficiently distribute energy and power among different sources in EV so that the life of EV is increased which in turn propel the people to purchase EV in the near future. The study will be based on conditions of Asian countries such as road conditions, economic conditions and general mindset of people. This research can be further implemented in real time EV or in industries producing EV on market scale.
.png)
Block Diagram of EMS of EV.
EV Configurations
To increase EV life cycle, limit surge discharge and system efficiency, it is important to combine two or more energy sources in a proper topology which extracts maximum benefits from the source combination. The SC/battery combination offers different benefits such as drive range extension, peak power reduction, enhanced battery life and State of Health (SOH).
​
The combination of SC and battery is apt as it provides high power and energy density. Batteries combined with SC store energy at the time of braking and help in smooth transfer of energy. SC can easily sustain dynamic load profile and provides longer drive range therefore, SC/battery combination is adequate for dynamic power regulation and long life of EV.
​
The coupling between battery/SC combination and DC bus is applied through bidirectional DC-DC converters. Based on source and converter combination four topologies are popular such as
-
Passive parallel topology
-
Semi-active topology
-
Fully active hybrid topology
-
Series-parallel reconfigurable combination
EMS and strategies applied for power distribution in EV
.png)
EMS Classification of Hybrid Energy Storage System (HESS) in EV.
EMS in EV is the control system which helps to use the different energy sources judiciously. It is controlled by a microprocessor which receives input from sensors and power commands from control unit. After getting the power commands it initiates the energy transfer from battery to tires of EV. It also learns from previous experience and try to improve the drive range by the application of different control techniques.
To divide the power in effective and efficient manner the EMS uses many control algorithms which uses some input data such as driver command, trip length, SOC of battery and SC, speed of EV and generates the power distribution signal to meet the load demand while sustaining the charge of SC and battery, improving the efficiency and lifetime of EV.
Different techniques are investigated in literature for EMS in EV, they are broadly classified in two types
-
Rule based control strategy
-
Optimisation based control strategy
Objectives
-
To design an EMS which takes into account the road parameters such as realtime road slope, friction coefficient and mass entering/leaving the Electric Vehicle EV.
-
To develop an integrated system which takes into account the combination of road conditions and power loss for sharing of energy among different sources.
-
To investigate the various configurations of EV design on the developed controller and find the best design for the varying road conditions prevailing in real time.
-
To implement the entire system on hardware setup to test its applicability in real time situations.
Velocity prediction using Hybrid Artificial Neural Network
-
Velocity prediction is an integral part for the development of robust Energy Management System (EMS) of an Electric Vehicle (EV) which essentially enhances the performance and life cycle of the vehicle.
-
In this work an ANN based approach combining Back-propagation Neural Network (BPNN) and Radial Basis Function Neural Network (RBFNN) is used to forecast velocity on different prediction horizons.
-
The BPNN is used to predict the preliminary velocity and RBFNN is used to forecast the prediction error. Then the final velocity is obtained by adding the BPNN velocity values and RBFNN error values.
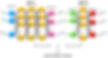
Results
-
These methods are tested on two conventional driving cycles viz. Manhattan and WYU driving cycle and one mixed cycle which is created by combining different random driving cycles.
-
Figures below show the comparison of predicted velocity and actual velocity for mixed cycle for horizon lengths of 2 sec, 3 sec, 4 sec, 5 sec respectively by using proposed method.
-
Results of proposed technique on Manhattan and WYU drive cycles are also shown below.
-
The results are studied in terms of Root Mean Square Error (RMSE) where the proposed network yields the least value in different prediction horizons as compared to conventional BPNN method.
For mixed drive cycle

Velocity prediction by proposed method for 2 sec

Velocity prediction by proposed method for 4 sec

Velocity prediction by proposed method for 3 sec
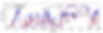
Velocity prediction by proposed method for 5 sec
For WYU and Manhattan drive cycle
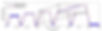
Velocity prediction by proposed method for 5 sec for WYU cycle
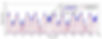
Velocity prediction by proposed method for 5 sec for Manhattan cycle
Courses taught during PhD
PMRF Deliverables
-
DSP Lab - Gauhati University (Nov 2021)
-
Microprocessor - Course Instructor - Gauhati University (Jan-May 2022)
-
Signal and system- Course Instructor - Gauhati University (Aug 2022- Dec 2022)
-
Microprocessor - Course Instructor - Gauhati University (Jan 2023- June 2023)
-
Signal and system Lab- Lab Instructor - Gauhati University (Aug 2023 - Present)